The Carter Catastrophe
A Bayesian argument for why humans will soon be extinct
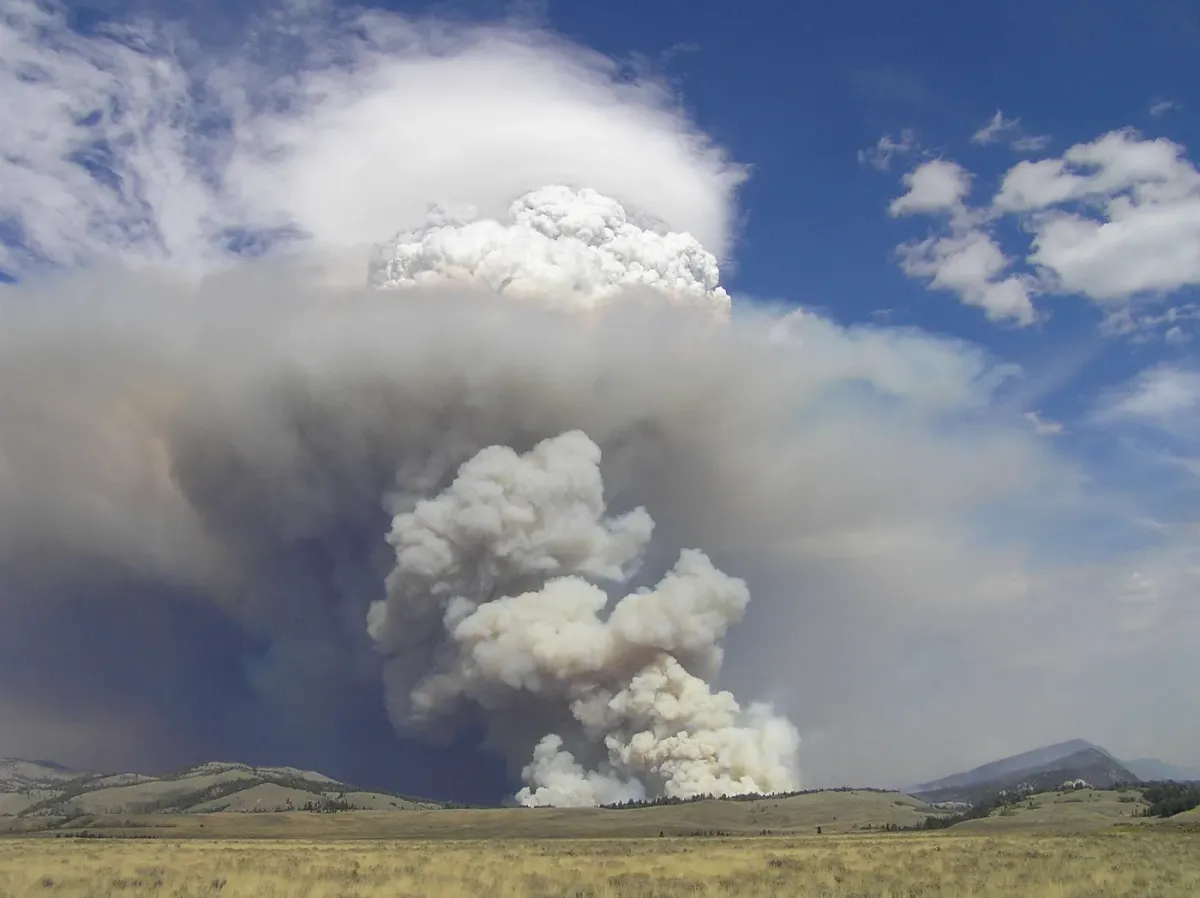
I first encountered The Carter Catastrophe in Manifold: Time by Stephen Baxter. It presented a fascinating probabilistic argument that humans will go extinct in the near future. It is called the Carter Catastrophe because Brandon Carter first proposed it in 1983.
I decided to double-check it, and it turns out to be mathematically sound. Here’s the argument.
Bayesian Basics
The argument is based on Bayesian analysis, so I’ll go over the fundamental facts we’ll need in this section before making the argument.
First off, notationally we’ll say P(A|B) is the probability of A being true given the fact that B is true. The most common example is something like A is the event of having a disease and B is the event of testing positive for that disease.
As long as there is such a thing as the test giving a false positive, then P(A|B), the probability of having the disease given that you tested positive for it, is not 100%.
Bayes’ Theorem is a formula for computing probabilities of this type:

I’ll sometimes refer to P(A) as the prior probability or just “prior,” because it represents our belief in A being true before we found out the new information of B. In the language of the above example, we’d say what’s the probability you have the disease before we knew you tested positive for it?
Bayes’ Theorem is a mathematical theorem that is not disputed, but it often produces surprising results that are hard to believe. We should trust those results because humans are pretty bad at judging probabilities.
For example, suppose the test for a disease is 99% accurate: has 1% chance for a false positive and 1% chance for a false negative. Suppose also that the disease only occurs in 0.5% of people.
If you test positive for the disease, then you might think there’s a 99% chance that you have the disease. But when you plug the numbers in, you’ll find that you actually only have a 33% chance of having the disease. A good doctor will retest you, realizing that it was likely a false positive.
The counterintuitive thing going on here is that Bayes’ Theorem took into account our prior knowledge that it was extremely unlikely that you had the disease coming in for the test: only a 0.5% chance.
If I just ask you a simple question, you’ll understand why Bayes’ Theorem told us what it did. What’s more likely: having the disease or having a false positive?
The answer is simple: having a false positive (because there’s a 1% chance instead of a 0.5% chance). Bayes’ Theorem computed exactly how much more likely.
An Illuminating Example
Let’s start with a basic example.
We have a giant tub of golf balls, and we can’t see inside the tub. There could be 1 ball or a million. We’re told the owner accidentally dropped a red ball in at some point. All the other balls are standard white golf balls.
We decide to run an experiment where we draw a ball out, one at a time, until we reach the red one.
First ball: white.
Second ball: white.
Third ball: red.
We stop.
We’ve now generated a data set from our experiment, and we want to use Bayesian methods to give the probability of there being three total balls or seven or a million.
In probability terms, we need to calculate the probability that there are x balls in the tub given that we drew the red ball on the third draw. Any time we see this language, our first thought should be Bayes’ Theorem.
Define Aᵢ to be the model of there being exactly i balls in the tub. I’ll use “3” inside of P( ) to be the event of drawing the red ball on the third try. We have to make a finiteness assumption, and although this is one of the main critiques of the argument, we can examine what happens as we let the size of the bound grow.
Suppose for now the tub can only hold 100 balls.
A priori, we have no idea how many balls are in there, so we’ll assume all “models” are equally likely. This means P(Aᵢ)=1/100 for all i. By Bayes’ Theorem we can calculate:

That might look confusing, but I encourage you to try it yourself. It’s literally just substituting in for each part of Bayes’ Theorem. There’s nothing suspicious here.
Note, for example, that P(3|A₁)=0 because the probability of drawing the red ball as the third ball given only 1 ball in the tub is 0. That’s why the summation starts at 3.
So, if the tub can hold 100 balls, then our experiment where we found the red ball on the third try tells us there’s around a 9% chance that there are exactly 3 balls in the tub.
That bottom summation remains exactly the same when computing P(Aₙ|3) for any n and equals about 3.69, and the (1/100) cancels out every time.
So we can compute explicitly that for n > 3:

This is a decreasing function of n, and this shouldn’t be surprising at all. It says that as we guess there are more and more balls in the tub, the probability of that guess being right goes down.
This makes sense because it’s unreasonable to think we’d see the red one that early if there are actually 100 balls in the tub.
Thinking about the example
There are lots of ways to play with this. What happens if our tub could hold millions but we still assume a uniform prior? It just takes all the probabilities down, but the general trend is the same:
It becomes less and less reasonable to assume large amounts of total balls given that we found the red one so early.
Hopefully, you believe this even if you haven’t followed any of the explicit calculations.
If there were a million balls in the tub, then it’s extremely unlikely that we’d accidentally pull out the red one as the third one. If there are only 5 balls in the tub, then it makes sense that we see the red one as the third one. That’s not unlikely at all.
You could also only care about this “earliness” idea and redo the computations where you ask how likely is Aₙ given that we found the red ball by the third try. This is actually the more typical way the problem is formulated in the Doomsday arguments. It’s more complicated, but the same idea pops out, and this should make sense.
Part of the reason these computations were somewhat involved is that we tried to get a distribution on the natural numbers. But we could have tried to compare heuristically to get a super clear answer:
What if we only had two choices “small number of total balls (say 10)” or “large number of total balls (say 10,000)”? You’d find there is around a 99% chance that the “small” hypothesis is correct.
The Carter Catastrophe
Here’s the leap.
Now assume the fact that you exist right now is random. In other words, you popped out at a random point in the existence of humans.
So the totality of humans to ever exist are the white balls and you are the red ball. The same exact argument above applies, and it says that the most likely thing is that you aren’t born at some super early point in human history.
In fact, it’s unreasonable from a probabilistic standpoint to think that humans will continue much longer at all given your existence.
The “small” total population of humans is far, far more likely than the “large” total population, and the interesting thing is that this remains true even if you mess with the uniform prior.
You could assume it is much more likely a priori for humans to continue to make improvements and colonize space and develop vaccines giving a higher prior for the species existing far into the future.
But unfortunately, the Bayesian argument will still pull so strongly in favor of humans ceasing to exist in the near future that one must conclude our extinction is inevitable and will happen soon!
There are a lot of interesting and convincing philosophical rebuttals, but the math is actually sound.