Flavors of Randomness
Implications of the Efficient-Market Hypothesis
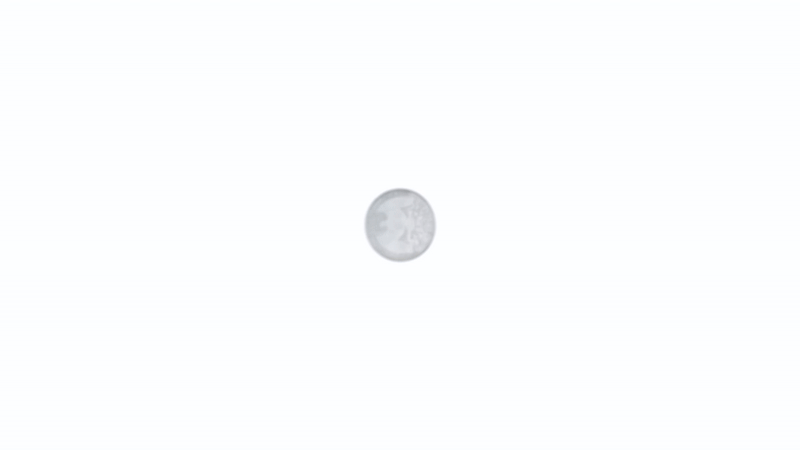
"The baseball announcer has it, of course, conveniently all wrong. Ted Williams is due to hit because he hasn’t hit for seven days. That’s red noise. Ted Williams is hot, he’s sure to hit tomorrow because he’s been hitting for seven days. That’s blue noise. The better description of an efficient market with random movements, which is white noise, is in between.” — Paul Samuelson
The above anecdote was made by economist Paul Samuelson in a 2004 interviewconducted by fellow Nobel Laureate Robert C. Merton for the American Finance Association (AFA). Lauded for his many contributions and often hailed as the ‘last of the great general economists’, the interview regarded one of Samuelson’s main contributions to finance, work on the so-called efficient-market hypothesis(Fema, 1965). This essay relates the implications of that work to the later development of the field of behavioral economics.
The Efficient-Market Hypothesis
The efficient-market hypothesis (EMH) states that asset prices fully reflect all available information in a market. That is, it should in theory be impossible to “beat” an efficient market consistently on a risk-adjusted basis. Given the ability to profit from private information, the conjecture assumes that rational investors are motivated to act by buying or selling an asset. In doing so, they reveal the implications of their analysis (of their private information), and so contribute to increasingly efficient market prices. In the competitive limit, market prices will therefor reflect all available information (both public and private) and prices will only change in response to new information. What one is left with, is the noise of investors’ decisions in unfounded information — which is considered to behave essentially randomly (see “Brownian Motion in Financial Markets”).
What Samuelson’s anecdote above and the efficient-market hypothesis (EMH) hence spells out is essentially a taxonomy of human interpretations of what may statistically be interpreted as random events. Events such as the usefulness of a price trend in predicting the future value of a stock or the dependence of a future base hit on a history of striking out or hitting streaks. His point is that people’s interpretations vary wildly, and that it goes against human nature to imply randomness by default, which in turn leads to fallacious expectations.
History
Prior to its popularization in the mid-1960, the roots of the efficient market hypothesis (EMH) can be traced back to Louis Bachelier’s 1900 doctoral dissertation Théorie de la spéculation (“The Theory of Speculation”). Bachelier was the first to use the theory of Brownian motion to describe stock and option market fluctuations as essentially random movements. His advisor at the University of Paris was Henri Poincaré and he successfully defended his thesis in 1900.

The key insight of Bachalier’s dissertation was the realization that if asset prices in the short term show an identifiable pattern, speculators will find this pattern and exploit it, thereby eliminating it. He argued, essentially, that as soon as the price of a stock or option begins behaving according to a predictable pattern, that pattern will disappear as speculators find and exploit it. The notes from Bachelier’s thesis defense committee, co-signed by Poincaré, describe the thesis in the following way:
One could imagine combinations of prices on which one could bet with certainty. The author cites some examples. It is clear that such combinations are never produced, or that if they are produced they will not persist. The buyer believes in a probable rise, without which he would not buy, but if he buys, there is someone who sells to him and this seller believes a fall to be probable. From this it follows that the market taken as a whole considers the mathematical expectation of all transactions and all combinations of transactions to be null.
Now considered the quintessential pioneering work in financial mathematics, the work was largely forgotten until being rediscovered in 1956 by Leonard Savageand put to the attention of Paul Samuelson, who arranged to have it translated by his colleague Paul Cootner. Samuelson used it to further develop Bachelier’s ideas and in 1965 publish two now momentous papers in finance: Proof That Properly Anticipated Prices Fluctuate Randomly (Samuelson, 1965a) and Rational Theory of Warrant Pricing (Samuelson, 1965b), both in Industrial Management Review. There, he proposed a model of option pricing closely related to the key thesis in Bachelier’s work, citing his influence.


A Random Walk Down Wall Street
Bachalier’s thesis argument is that asset prices are best modeled as a stochastic process, more specifically according to the theory of Brownian motion. A closely related idea, the random walk hypothesis, states that prices in an organizedmarket evolve at random, in the sense that ‘the expected value of their change is zero but the actual value may turn out to be positive or negative’. The term “Random Walk Hypothesis” was popularized by Burton Malkiel’s 1973 book A Random Walk Down Wall Street in which the author argues that because asset prices exhibit signs of random walk, investors cannot outperform market averages:
Famously, students were given a hypothetical stock that was initially worth fifty dollars. The closing stock price for each day was determined by a coin flip. If the result was heads, the price would close a half point higher, but if the result was tails, it would close a half point lower. — Malkiel (1973)
Malkiel gathered the results from his experiment in a chart and brought it to a technical stock analyst, who seeks to “predict future movements by seeking to interpret past patterns on the assumption that history tends to repeat itself”. The analyst told Malkiel that they needed to immediately buy the stock.
Random walks
Mathematically, a random walk is known as a stochastic or random process that describes a path that consists of a succession of random steps on some mathematical space such as the integers:
One-dimensional walk along the integers
Start with zero and for each step, move either +1 or -1 with equal probability. Put a marker on zero on a number line and flip a coin. Heads, you move it to 1 and tails you move it to -1. After five flips, the marker will either be on -5, -3, -1, 1, 3 or 5 because:- There are ten ways of landing on 1 (three heads and two tails)
- There are ten ways of landing on -1 (three ails and two heads)
- There are five ways of landing on 3 (four heads and one tails)
- There are five ways of landing on -3 (four tails on and one heads)
- There is one way of landing on 5 (five heads)
- There is one way of landing on -5 (five tails)
Mathematically, we can define such a one-dimensional random walk along the number line by taking the independent random variables Z₁, Z₂, …, Zₙ, where each is either +1 or -1 with a 50% probability of either. Then set S₀ = 0 and define the sum:

The series {Sn} is called the simple random walk on Z. The expected value of the sum, perhaps as expected, zero. Higher order random walks are plotted and estimated in similar ways.
Markov chains
A random walk based on integers is an example of a Markov process. Named after Russian mathematician Andrey Markov, a Markov process is the term used to describe sequences in which future events rely on events that have already happened, also sometimes called “memorylessness”. A Markov chain is a stochastic model describing a sequence of possible events in which the probability of each event depends only on the state attained in the previous event:
Flavors of Randomness
The flavors of randomness Samuelson describe, of white-, red-, and blue noise, can essentially be summarized as three ways of thinking about what will happen in the future, given what we already know has happened. Taking the efficient-market hypothesis as given, they describe how one might go about thinking about the random behavior of e.g. asset prices in an economic market or base hits in baseball.
White noise / True Randomness
“White noise is truly a random walk. The future is independent of the past. Knowing that the stock rose yesterday has no influence on the probability distribution of what it will do percentage wise between today and tomorrow. That’s white noise. Zero serial correlation coefficient in statistical parlance.“ — Paul Samuelson
Randomness interpreted as white noise considers events as truly random and independent of past events. That is, a white noise interpretation reflects the view that, despite the fact that a stock has gone up every single day in the last three months, tomorrow is a new day and its former price movements provide us with no better approximation about its future behavior than would a coin flip. The white noise interpretation of randomness makes explicit the fundamentally unintuitive notion that despite the very low probability that 76 coin flips in a row will turn up heads (0.0000000000000000000013%), after 75 coin flips, the odds of the 76th coin flip turning up heads is still 50% because the coin has no memory.
Red noise / “The Gambler’s Fallacy”
“Red noise (is what) I called regression towards a mean, where there is a negative serial correlation through time. So that if things (went) up a lot yesterday, today you should bet that they won’t go up as much as they would normally do between today and tomorrow.That is red noise. “ — Paul Samuelson
The “pessimistic” interpretation of random events, red noise, also known as the Gambler’s fallacy, is the mistake notion that if something happens more frequently than normal during a given period, it will happen less frequently in the future (or vica versa).
In literature, descriptions of the fallacy trace as far back a 1796 story entitled A Philosophical Essay on Probabilities by Pierre-Simon Laplace, in which he describes the ways in which men calculated their probabilities of having sons:
"I have seen men, ardently desirous of having a son, who could learn only with anxiety of the births of boys in the month when they expected to become fathers. Imagining that the ratio of these births to those of girls ought to be the same at the end of each month, they judged that the boys already born would render more probable the births next of girls."- Excerpt, "A Philosophical Essay on Probabilities", Laplace (1976)
Blue noise / “The Hot Hand Fallacy”
“Blue noise is the opposite. If things went up yesterday, they will go up, in a probability sense, more often tomorrow. “ — Paul Samuelson
The more “optimistic” interpretation of random events, blue noise, also sometimes known as the Hot hand fallacy, is the phenomenon that a person who experiences a successful outcome has a perceived greater chance of success in future attempts. Purported to have been disproved (as a real-world phenomenon) in a 1985 study by Tversky et al (1985), more recent studies have indicated that future performance might not necessarily be unrelated to a previous “hot streak”.
Regardless, the interpretation of truly random events as indications of a higher probability of certain future events still remains a fallacy.
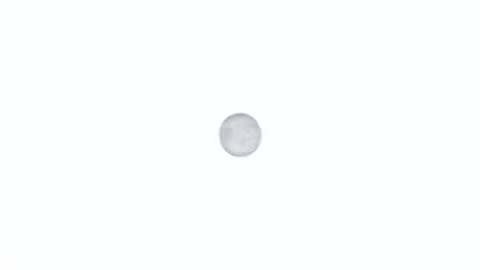
Epilogue
Samuelson’s work on the efficient market hypothesis (and in turn his descriptions of how humans misperceive randomness) is one of many works in neoclassical economics whose lacking accuracy in their predictions laid the groundwork for what later became the field of behavioral economics. Behavioral economics is the study of the effects of psychological, cognitive, emotional, cultural and social factors on the economic decisions of individuals and institutions and how those decisions vary from those implied in classical theory. For more information, I recommend the book “Thinking, Fast and Slow” by Daniel Kahneman.