How Mathematical Proofs can Help Unlock the Secrets of the Brain
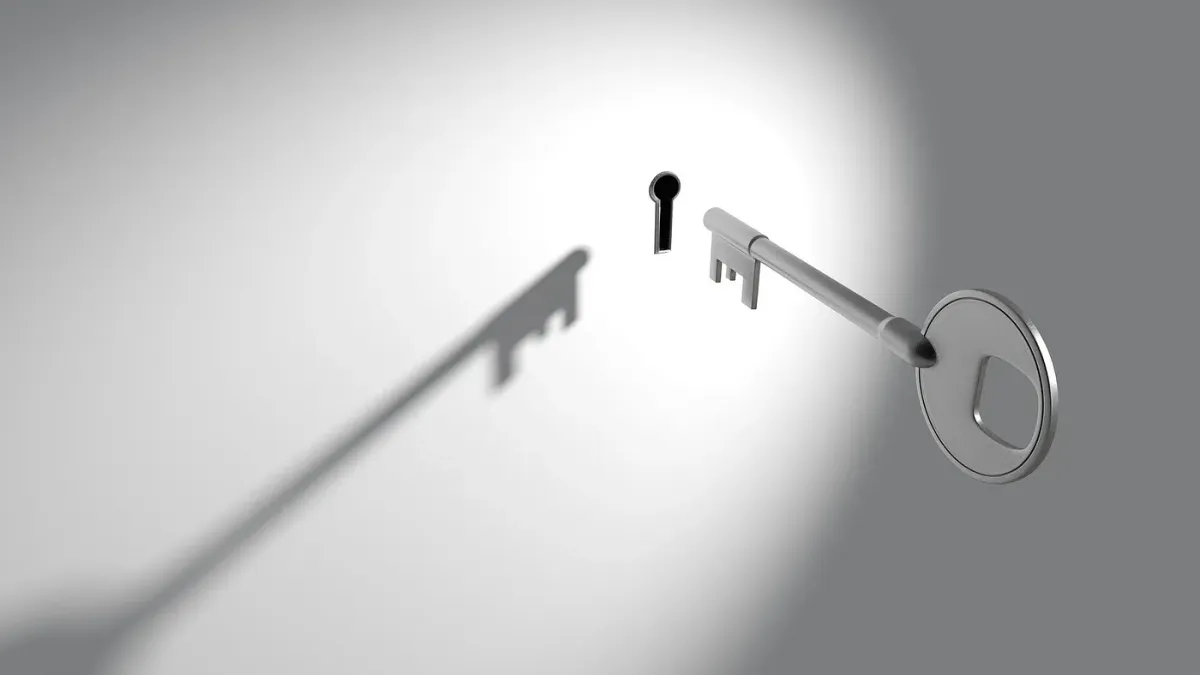
Computational neuroscience, broadly defined, is the mathematical and physical modeling of neural processes at a chosen scale, from molecular and cellular to systems, for the purpose of understanding how the brain represents and processes information. The ultimate objective is to provide an understanding of how an organism takes in sensory information, how information is integrated and used in the brain, and how the output of such processing results in meaningful decisions and behaviors by the organism in order to allow it to function and thrive in its environment. In an attempt to understand what the brain is doing and how, we build computational models that aim to replicate and explain the observed or measured data in order to arrive at a deeper understanding of brain function.
The process goes something like this: Beginning with a set of experimental observations or measurements, for example measuring the electrcial activity of neurons in response to a stimulus (electrophysiology), a model is postulated. The model aims to provide a set of rules or relationships that if given the initial experimental observations (or at least a part of it) would be able to describe and explain some aspect of the brain. In general, this almost always begins with a qualitative “guess” about how the data fit together and what are the likely rules that govern the relationships between it. This qualitative picture of the model is then “translated” into a quantitative mathematical framework The model, once constructed, is still nothing more than a guess, and so testing it with the goal of building circumstantial support for it (or against it) is then carried out by numerical (computer) simulations, often where the answers or outputs are known from experiment, so that they can be compared with the outputs computed by the model.
But what if we define mathematical neuroscience not as the generation of hypotheses based on numerical simulations of postulated ideas, but as the systematic analytical investigation of data driven theorems?
At this point several outcomes are possible, assuming the model is at least partially correct. One possibility is that the model is able to describe the data set used to constructed it but cannot make any novel non-trivial predictions or new hypotheses. A more productive outcome is when the model results in a unexpected experimental hypothesis that can be tested and verified. This may lead to novel experimental findings. In turn, new data then allow the fine tuning or modification of the model in an iterative way. But in all cases though, the core of the process is the same: one guesses at a model and uses mathematics to justify the guess. The actual validation of the guess is based on numerical simulations, and an iterative approach improves the model. Note however, that in the typical way in which computational neuroscience is practiced, the mathematics involved is primarily descriptive and does not participate in the process of discovery. Given this, we can define computational neuroscience, somewhat more provocatively, as numerical simulations of postulated models and ideas constructed from qualitative hypotheses or guesses.
But what if we define mathematical neuroscience not as the generation of hypotheses based postulated ideas, but as the systematic analytical investigation of data driven theorems? The key ideas is that mathematical conjectures about the brain can be written down and logically proven. The axioms, i.e., the starting point ground truths, are not unknown or postulated hypotheses about how the brain might work, but, within the limits of experimental verification, are the simplest possible set of experimentally verified ‘knowns’ that support the construction of the statement of truth being made by the conjecture. In other words, the initial goal is to set up a conjecture that is mathematically sound and is based on an agreed upon set of experimentally validated axioms about the neurobiology, and then use any mathematics possible to formally extend the starting point in order to arrive at a novel insight or hypothesis or new understanding about how the brain works.
These axioms are the same types of experimental observations and measurements that form the starting point in computational neuroscience, but instead of qualitatively guessing a possible relationship that explains the set of observables, the objective is abstract, the set of experimental observations into a corresponding complimentary set of simple mathematical statements. No assumptions or guesses whatsoever regarding the relationship between either the set of experimental observables or their corresponding mathematical representations need be made at this point. Only, as simply as possible, straight forward statements of fact that everyone would agree upon.
The next step is to set up a conjecture that says something about the set of axioms. While this is itself a guess, often the product of much trial and error, it is a mathematical guess. This means that once a plausible conjecture is written down, it can be attempted to be proven. This is the fundamental consideration that differentiates computational neuroscience from mathematical neuroscience as I’m defining it here. In computational neuroscience a model is written down that is a guess about the relationship between the data itself, but there is no formal logical way to “prove” the model correct or incorrect. So numerical simulations are done. But this is never proof of anything. Writing down a valid conjecture on the other hand is a very narrow statement about a very specific set of facts. And it has the potential to be proven; meaning that it can be established as true or false analytically. And once proven it is true forever. One has established a truth about the relationship between the starting axioms from a logical set of arguments. No simulations or other guesses are required.
Combine such a mathematically rigorous approach with emerging applications of machine learning to neuroscience discovery …
As a proof of concept and power of this approach, in our own research we did just this. A mathematical description about how neurons and networks of neurons communicate with each other led to a series of mathematical proofs that resulted in a theoretical framework and prediction about how there must exist a balance between the time an individual neuron takes to process information internally, i.e. locally, versus the amount of time it takes for the information to propagate throughout the network, i.e. globally. (What we called the refraction ratio.) This, in turn, led to an experimentally testable prediction about how real biological neurons optimize this mathematical ratio. We were able to show computationally that at least in one specific type of neuron in the brain, the cells have shapes (morphologies) specifically designed to nearly preserve the theoretically predicted ideal ratio. This was not a serendipitous discovery about the neurobiology. We didn’t just get lucky. The mathematics and theoretical work pointed us in that direction.
Like most other areas of science, there is a tremendous and continuously growing amount of data in neuroscience, but comparatively less theory about how all the data comes together in order to arrive at a sytems-like engineering understanding about how the brain works. In fact, the link between mathematics, engineering, and neuroscience will only continue to become ever more stronger. It has to. We simply will not be able to understand how the brain works as a system without it.
Combine such a mathematically rigorous approach with emerging applications of machine learning to neuroscience discovery, and we could be on the verge of understanding the brain, and how it fails in disease, beyond anything we could have imagined so far.