Here’s What a Theft Taught me about Statistics and Probability
A statistics lesson that can help you protect your valuables
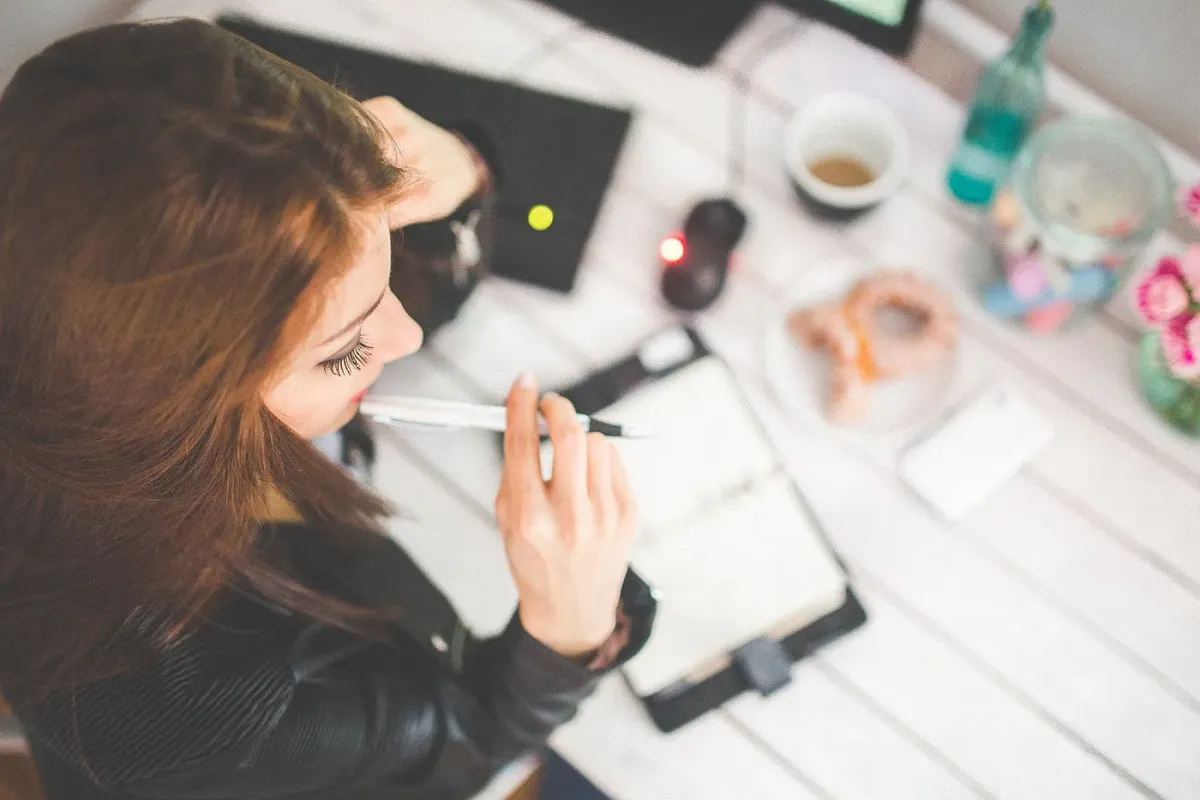
The field of Statistics and Probability is useful for a lot of things like weather forecasts, scientific research, machine learning, and data analysis. However, I recently had an experience which caused me to add one more thing to that list: guarding your laptop against theft.
In the month of September last year, I suffered the same fate as many people around the world. My laptop got stolen. To be honest, the incident was mostly my fault. I made a hasty decision based on my understanding of statistics, but after I lost the device I learned a lesson about statistics that could have saved it.
In this article, I try to explain the kind of statistical thinking that caused me to err as well as the lesson I learned from it. That lesson is from the field of Discrimination and Classification.
Discrimination and Classification
Discrimination and classification are closely related concepts in the world of Statistics and Probability. For those who are conversant with topics in machine learning, this would be familiar. In their book Applied Multivariate Statistical Analysis, Johnson and Wichern state, “discrimination and classification are multivariate techniques concerned with separating distinct set of objects and with allocating new objects to previously defined groups.”
In plain English, this means that if we are presented with certain “objects”(which could be things, scenarios or people), we would like to put those objects into different groups based on certain characteristics. As an example, if I were to present you with detailed information(like income, level of education and morality) of a bunch of strangers, discrimination could help you distinguishbetween trustworthy and untrustworthy people among them. Based on the groups you’ve made, if I present you with a new stranger, classification would teach you where to allocate that new individual.
This is the same idea that insurance companies might use to classify someone as being likely or otherwise to be in an accident soon. This would inform the amount of premium that customer might be made to pay.
This was the kind of knowledge I needed last year. Now that I have that knowledge, I realize that if I had discriminated and classified properly, I would still have that laptop today.
So what happened on the day of the theft?
Discriminating and Classifying The Laptop
When I lost my laptop last year, I was a final year student of mathematics and statistics in the university. On that September day, I was in hurry to get to the lecture hall and was in no mood for any delay. I was running late for an interim assessment that was about to start in the next 10 minutes, but I needed a place to keep my bag which contained my laptop.
If I wasn’t so late, I would have readily gone to keep my bag at the usual place I kept it. However, time wasn’t on my side, so I began to hesitate on that decision.
Should I keep it in the safe place I trust? Or should I send it into the lecture hall and place it where everyone else places their bags? I had heard of people’s bags getting stolen during exams but I thought perhaps I just might be lucky.
In order to make the best decision based on convenience and safety, I turned to the one thing I knew best apart from math: statistics and probability. I was a student of statistics, after all.
So I asked myself, “What is the probability that my laptop would get stolen if I keep it in the usual safe place?”
I replied to myself, “Obviously, close to zero. The place is very safe.”
“What about where everyone else places their bags?”
And that’s where I failed my statistics lecturers in the answer I gave. The convenience of not having to walk far in order to store my bag clouded my sense of judgement.
I knew that my laptop was attractive and very expensive. Many of the students in my class had seen it before and were probably envious. That should have informed me that the probability of it getting stolen was higher than usual.
Yet, I managed to convince myself that everything would be fine. I was certainly going to keep one eye on my test paper and keep the other eye on the bag. Because of the intricate plans I had, I reasoned that the probability of me losing the bag was close to zero.
Well, I failed. The thief had no regard for probability at all.
However, in the next semester, I took the course Multivariate Analysis and once I studied Discrimination and Classification, I understood what I had done wrong.
Discriminating and Classifying The Right Way
Here’s how my past self should have reasoned about the situation. What I needed to realize was that once I strip away all the unnecessary details, this is really all just a problem in Discrimination and Classification. What my past self was trying to do was to classify my laptop into one of two categories: likely to get stolen or not likely to get stolen.
Now, before we go through the proper way to classify my laptop, we need to define some variables. Let
- x = my laptop
- S = collection of all stolen laptops in the world
- N = collection of all non-stolen laptops in the world
- f = probability density function associated with S
- g = probability density function associated with N
- c(S|N) = cost of classifying the laptop in S when it actually belongs to N
- c(N|S) = cost of classifying the laptop in N when it actually belongs to S
- p = prior probability of S
- q = prior probability of N
Now that we’ve got all that notation out of the way, we need to determine which group my laptop is likely to fall into based on information we know. Is x(my laptop) likely to fall into group S(stolen laptops) or group N(laptops not stolen)?
To classify appropriately, Johnson and Wichern have provided us with formulas in their book that we can work with.
Classify x into S if

But classify x into N if

For the purposes of this article, the most important parts of those equations are the prior probabilities(namely, p and q) and the costs of misclassification(namely, c(S|N) and c(N|S)).
Prior Probability
Prior probability of a group of objects can be regarded as the proportion of objects that typically fall into that particular group. So in the case of stolen laptops, the prior probability of the group S is the proportion of laptops in the world that get stolen. In order to know how likely it is that a random laptop falls into this category, we need some official statistics.
One such useful statistic comes from a 2018 Kensington report (PDF), which suggests that 1 in 10 laptops will likely be stolen or lost from an organization over the lifetime of each computer. So if I were to take this value as it is and apply it to my situation, that puts p = 0.10 and q = 0.90. (And for those of you who are more curious, Techspective cites a Gartner study indicating that a laptop is stolen every 53 seconds.)
However, you might look at the probability 0.1 and say, “Bro, that’s so small! There’s no way your laptop is getting stolen.” But that’s where I got it wrong. Prior probability isn’t the only thing needed to do a proper classification.
Cost of Misclassification
Cost of misclassification means exactly what you think it means. It accounts for the cost, mostly financial, of making a wrong classification of an object. In other words, it is the cost of classifying an object as belonging to one group when it in fact belongs to a different group.
So in the case of stolen laptops, we could take into account the cost of classifying my laptop as not likely to be stolen when it is actually likely to get stolen. That is what I failed to think about.
If I classified the device as not likely to get stolen but it eventually got stolen, what would be the cost to me?
To answer that question, I should have realized that I was a student who had managed to squeeze out some money to buy a laptop like that. The cost of losing such a laptop in the middle of a busy school year was going to be immense. So although 0.1 might have seemed a small probability, the cost of several hundred dollars should have been enough to knock some sense into me.
Nevertheless, I could have also considered the cost of classifying a laptop as likely to be stolen when it is actually not likely to be stolen. However, that cost should be irrelevant, since “it is better to be safe than sorry.”
Johnson and Wichern said it best. “Another aspect of classification is cost. As an example, failing to diagnose a potentially fatal illness is substantially more ‘costly’ than concluding that the disease is present when, in fact, it is not.”
They continued, “An optimal classification procedure should, whenever possible, account for the costs associated with misclassification.”
Thanks, Johnson and Wichern. I wish you both had told me this while I still had my laptop.
Conclusion
Like I said at the start, statistics and probability are useful for a lot of things: weather forecasts, scientific research, machine learning, data analysis, and guarding your laptop from thieves. Ultimately, statistics helps to make better decisions. That is what this field of study was made for.
From the littlest of decisions like placing your bag down, to the biggest of decisions like solving unemployment, statistics has the tools to supply you with probabilities you can work with.
Now, whenever I take a decision, I ask myself what the cost of misclassification is. Is the decision I’m about to make worth the risk? No matter how small the probability is, am I about to make a decision that I would deeply regret later if it goes wrong? If the answer is a confident YES, then I stay clear of repeating my past mistake.
So in a sense, the theft of my laptop has taught me a lesson about Probability that sounds counter-intuitive: in the face of high cost, Probability says ignore probability.That’s a rule I understand very well now. It took a thief to teach me that.
REFERENCES
[1] Richard A. Johnson and Dean W. Wichern. Applied Multivariate Statistical Analysis(Sixth Edition). Pearson, pp 575–584.
[2] Kensington, Survey: IT Security & Laptop Theft, 2016